|
Home > Articles > Neural Networks > Character Recognition
Introduction
Character recognition is one of the areas of AI which has been developed for many years and still has many things to be done about it. I've read somewhere that one of the best neural networks had a success of about 98% on some testing patterns and a human is 99% correct on the same patterns, which means that the people are missing something which makes them more efficient. This network was very complicated, had many filters, centering of patterns, rotation, scaling and other things manipulating the character, but the thing that caught my interest was its ability to look at the context. We also do this but without noticing it. It's become mechanical job of the brain. For example: You look at a handwritten word of five characters like "house" and you can read all the characters except the second one, because it seems something like "c" or "e". A human knows the surrounding letters and through them he'll know what the missing letter is, because there aren't much words with the mask "h*use". A human does not recognise a word letter by letter. This fact makes us so good at this. The network I mentioned above had this ability and it reached a very high percent of success, but still something is missing. I think this is the ability of humans to understand the concept for writing individual letters. For example when I ask you how the letter "a" is written you will answer "A small circle with a short (curved at the bottom) line on the right touching the circle". People don't care how the circle is written, they just find something that looks like a circle, and if they find the short line on the right they say - "This is an "a"". We are better that them. There will be times however when they will be better than us!
Hebbian Links
A network using Hebbian links is designed to differentiate one situation from another. Their answer of a question can be "Yes" or "No". That's why they are asked questions which can be answered with "Yes" or "No". I'll give an example: You learn one such network how does a sad face look and how does a happy face
looks (look at Figure1 - the white boxes can be represented with 0s and the grey ones with 1s). Then you got one face pattern, which is damaged (For example - Figure
3), and you present it to the network. It answers
whether it looks happy or sad.
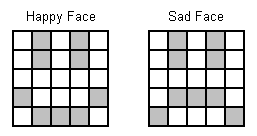
Figure 1
The interesting in hebbian links is that they remember the differences between the two situations. A learnt network wouldn't pay attention to anything but the mouth. And that's very logical - you can't say looking only
at the eyes whether the face is sad or happy. A network like this has N input neurons and an output neuron. Each input is connected to the output with an
edge, which has its own weight. I will not explain the learning process in details, but it is something like this: You get two
or more learning patterns divided into two groups. The first group we associate with the number 0 and the second group with
the number 1. These will be the outputs, which we will want when we ask the network for a presented test pattern. If the
network says 0 then it thinks the pattern is from the first group, and if it says 1 then the pattern is more likely to be
from the second group. In the learning process the weights of the edges are increased or decreased depending on the desired
output. If we feed the network with a pattern from the first group we will give it also the desired output which is 0. The
weights are being increased for the one group and decreased for the other. It is normal that the values for the edges
connected with the eyes of the face will stay close to 0, because the one image will increase them and the other will
decrease them. But the edges around the mouth will be totally different. Some of them will be positive, while others will be
negative. The weights will look something like this. (N: neutral, not used, 0: around zero, +: positive, -: negative)
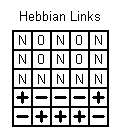
Figure 2
When we feed the network with an input it sums the positive weights and the negative weights. It outputs the output
corresponding to the higher sum (for the negative is taken its absolute value). Example:
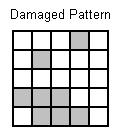
Figure 3
This test pattern will be considered as a happy face because it has more pluses than minuses. That's how hebbian links work
in general. I will not explain it further, because I think you can find more information about
them by yourself.
Character Recognition
Hebbian links and this faces was the first that I did when I started learning about AI. I asked myself how can I make them
recognise more than two groups of patterns, and here I ended up with the conclusion "With more than one network!". In the
English alphabet there are 26 letters. We can learn a hebbian links network for each pair of them. So imagine that we have
these networks, which are actually (26*25)/2 = 13*25 = 325. We give individual name to each one of them - for example a
network which is learnt for recognising whether a character is "a" or "k" will have the name "ak" (there is no network called
"ka", because there is no need for creating the same network). We now build the following structure.
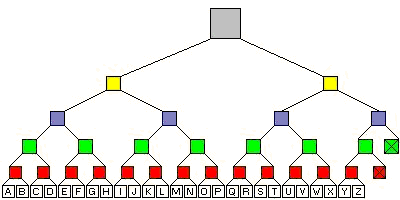
Figure 4
In the tree above we have four processing
levels - red, green, blue, yellow and grey. We will call them level 1, level 2,
level 3, level 4 and level 5 respectively. On the first level we have 13
individual hebbian networks for recognising the sequential letters, and we add
one more "virtual" output (the boxes with "X") which is
actually a random letter. This adding is done because there aren't enough
letters to be leaves of the binary tree. Each network is learnt for recognising
two individual letters, so every network on level 1 will output one letter. It's
like a football championship - two letters compete, but only one continues on
the competition. The outputs of the first level are then given as inputs of
level 2. The procedure is then repeated. For an example we have one letter which
we want to recognise: We present it to every network on level 1. Then we run
each network and it produces its own output. Then these outputs are used to
determine the exact networks on level 2. If we have the letter "c"
then we present it to the first network of level 1 and it will most likely
produce the output "b". Then we present it to the second network and
it should produce the output "c". Now we know which network we will
use as first on level 2 - the "bc" network. It will recognise the
charachter. This then goes up and up until the last network on the top is
reached. It should produce the result of the whole thing.
Conclusion
This championship thing works, I've tested it, but it's not good
enough. On my handwriting it has around 55-60% successive guesses. But with
centering the patterns and some other cosmetic things this percent could be
increased. In this article I just wanted to tell you how you can make the easy
for implementation hebbian networks work for more than two groups of patterns.
It may become something like multilayer perceptron but is somehow different. On
the first look it seems a little hard to implement but if you try it and
organise your sources well you'll do it. I made arrays of characters and others
stuff which identify each network. Then I run the desired level and the outputs
(which are also characters) I give to the upper array. I will not tell you how
to program because it's not actually my business. Well that's it for this
article and keep recognising!
Article content copyright © Stephen Tashev, 2003.
|
|